- Timeline: 2019 — 2020
- Skill demonstrated:
- UX Research
- User experience design
- User interface design
This is the story of how searching for published content was reimagined to become more contextual.
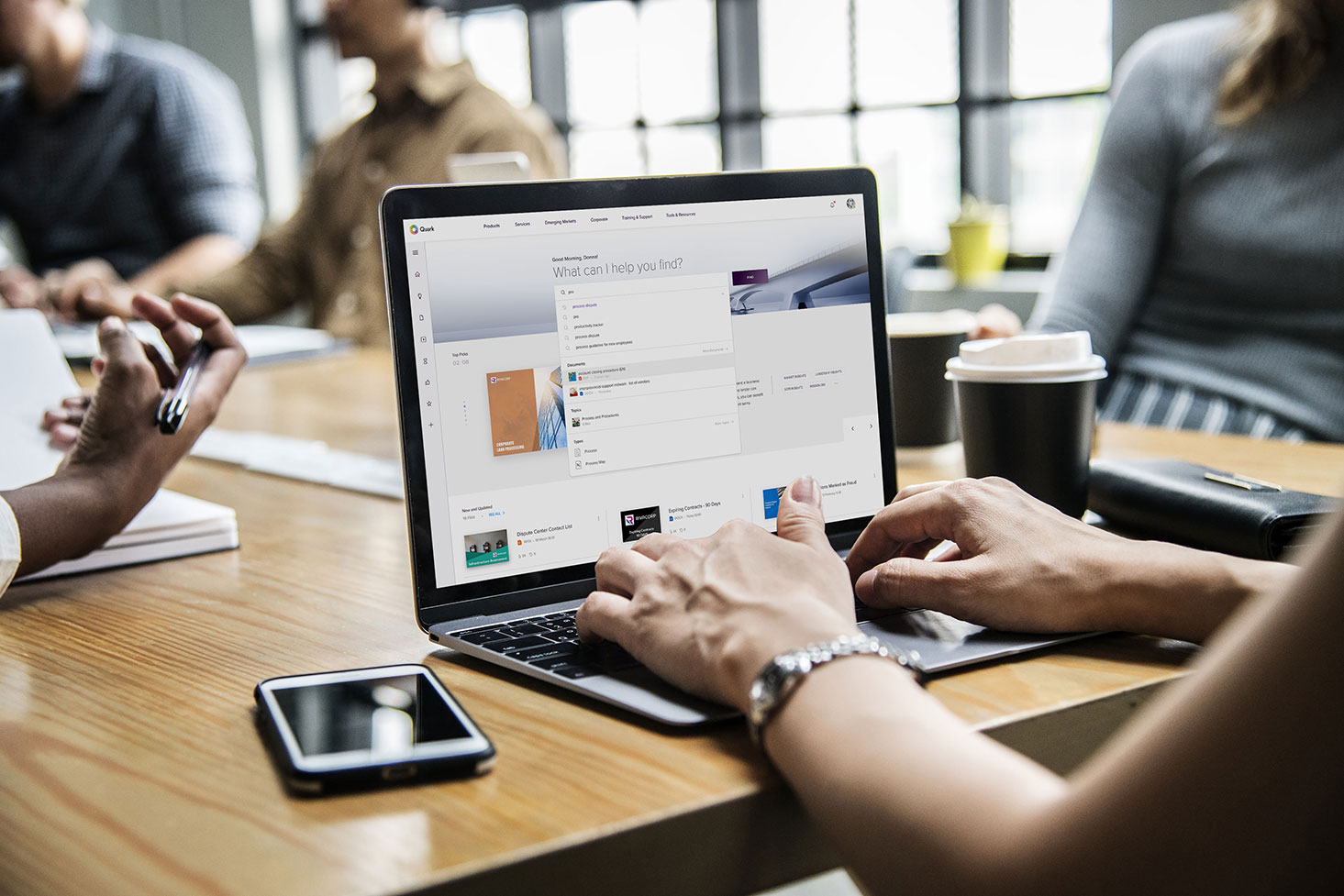
As the demand for content grows, so does the challenge of finding the right content, suitable for the right audience, and accessible at the right time.
A powerful system that helps knowledge workers access content on-demand should allow searching on more than just the obvious. It should also search within an assortment of metadata such as tags, descriptions and CMS based properties, and map the query to the context of the content type as well as the curent workflow to adjust the search recommendations accordingly.
Results
Additional metadata for search paremeters
To make searching effective for knowledge workers, the search mechanism includes additional metadata such as tags, descriptions and CMS based properties.
Improved contextual search
Searching within a certain view would priortize content within that context before searching on additional paremeters.
Support for power-users
It is now possible for power-users to search specifically within a particular search paremeter.
About the project
One of Quark's acquisitions of 2017, Docurated, now known as CE Access after the launch of Quark's Content Enablement Platform empowers teams to consume the right content at the right time and for the right audience. Whether to be viewed internally, such as training manuals and procedural documents to onboard new employees; or to be shared externally, such as pitch decks and demo videos to power sales and marketing enablement.
Problem
In a large scale organization with dozens of taxonomies and hundreds of documents published each month, the ideal way to find the right content would be to let the system help you. However, the search mechanism in Docurated lacked a lot of key functionality that users have come to expect from a modern search experience.
Asking the right questions
To get a better understanding of how users would prefer search to function, I spoke with the Professional Services team which works directly with customers to offer custom solutions and enhancements above-and-beyond what the product offers out of the box. I also closely worked with the Engineering team to get clarity the technical feasibility of improving search. Some common questions that came up during those dicussions included:
- "What are users searching for?"
- "Can we search within taxonomies?"
- "Can we search based on properties?"
- "How do you design search to make finding content easy?"
User feedback
Request 1: Show multi-faceted suggestions
As I am searching for content, show me the top suggestions grouped by various facets and taxonomies.
This was a big missing piece when it came to the existing search. Multi-faceted search needed not just an update to the design, but also required a major technical enhancement to fetch search results for additional parameters.
Request 2: Show auto-suggestions
As I am searching for content, I would like the system to correct spelling errors and offer suggestions to improve the odds of a direct match.
This request very closely resembles a Google-like search experience that most users are used to. The expectation is that the system should be smart enough to understand a bad-query and do its best to correct the same.
Request 3: Show recent searches
As I am searching for content, I would like to see my previous search queries, with the ability to remove them, if necessary.
Once again, this request very closely resembles a Google-like search experience that most users are used to, and rightly so: Much of a knowledge worker's day is spent researching topics that may seem repetitive. If a user has searched for something once, chances are, they might want to see it again.
Reframing the problem
Docurated was built over 10 years ago, when dynamic real-time suggestions weren't really a common practice. In 2019 however, users expect such functionality.
Quark's customer base had used similar experiences in countless other platforms and have seen the value first hand, so it was logical to research how other solutions that handle large multi-faceted datasets were using search suggestions to make finding content easier.
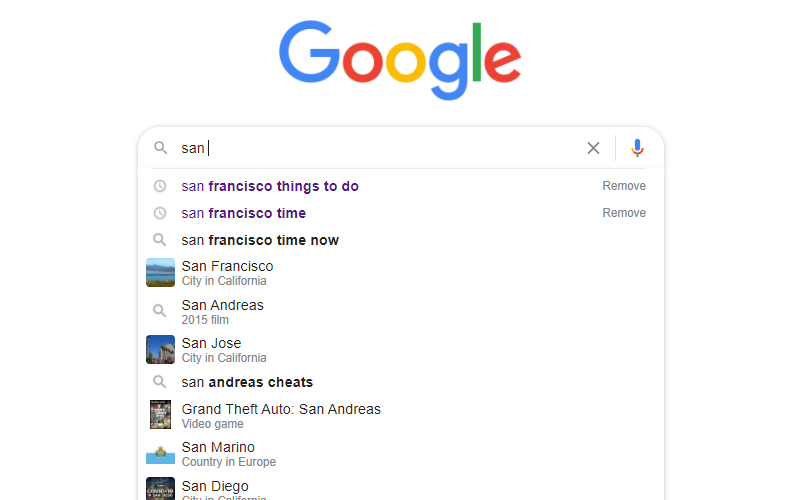
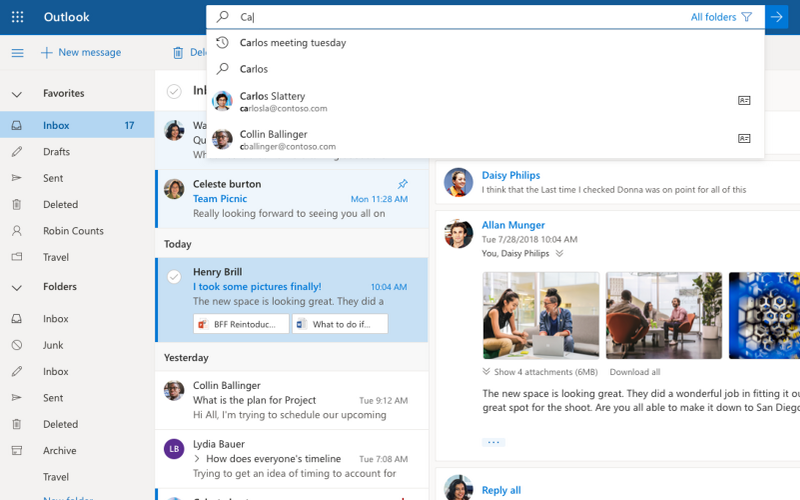
Solution
The biggest motivation for a redesigned search experience was to introduce a highly targeted facet-based search system.
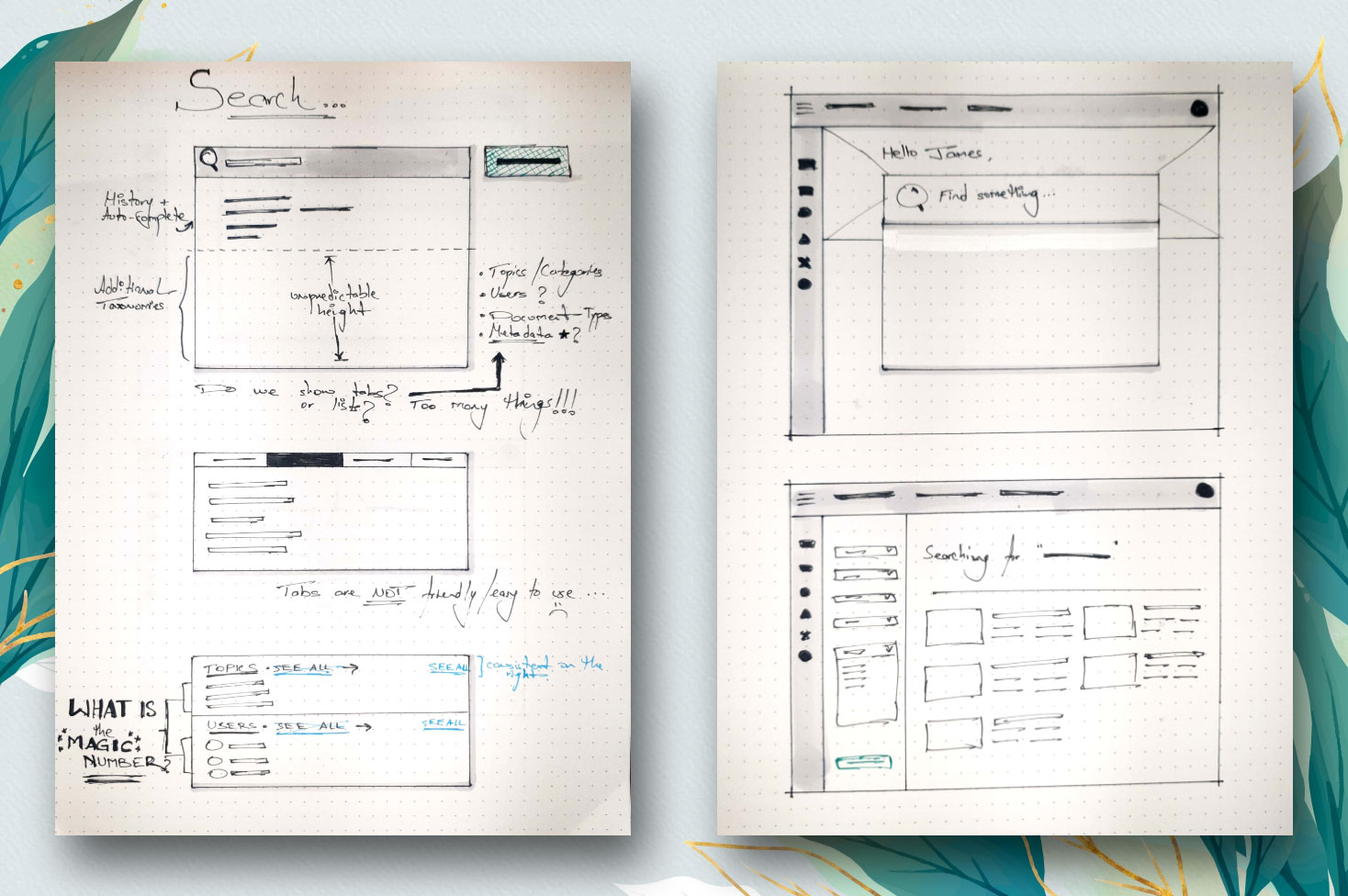
The multi-faceted search finds content across all taxonomies, sorting the suggestions into their respective groups. These groups are then ordered according to a prioritization system that’s contextual to where the user is at the moment, all inside a dynamic mega-dropdown.
The second most important addition to the search system was the introduction of property-based searches. If the search query matches an existing property, the user is able to search directly within that property. For example: "Modif…" would offer the suggestion of "Modified by", which would allow the user to search for people who may have modified a document.
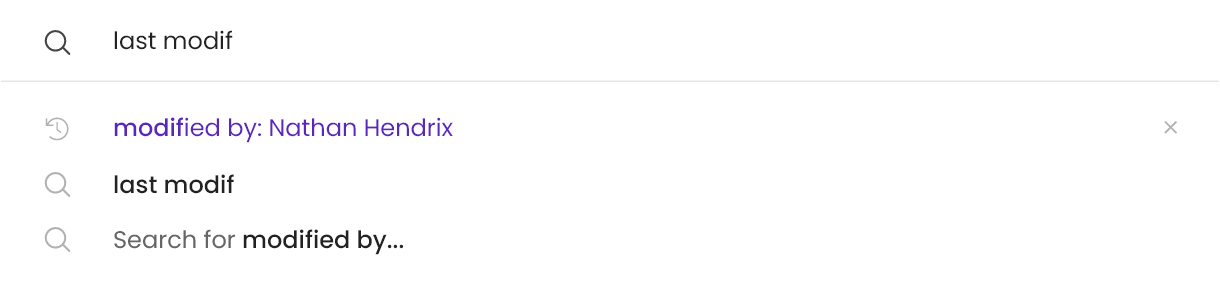
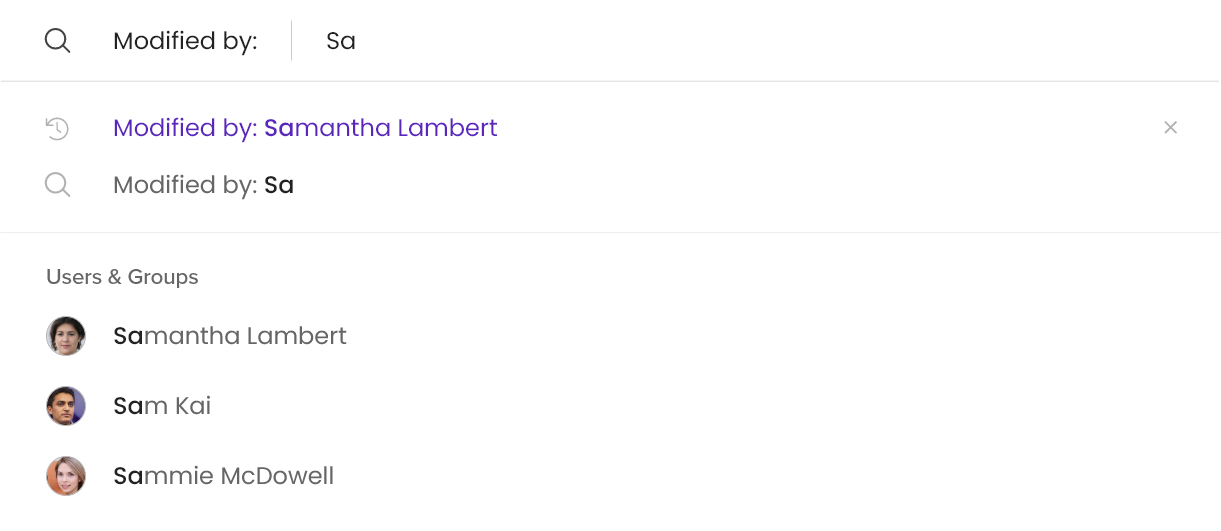
A great example of this sort of experience is what Google Chrome calls as "Tab to search", where typing "youtube [tab]" directs your search to Youtube instead of Google.
The dropdown also includes additional enhancements such as auto-correction and recent searches.